This new paper, published in Nature Reviews Cardiology, reports findings and guidelines from a workshop conducted by the National Heart, Lung, and Blood Institute. The workshop aimed to identify the knowledge gaps and the opportunities for future research on using the eye as a window to systemic disease, including developing a roadmap for the use of retinal imaging biomarkers for cardiovascular disease risk prediction. The retina is well suited to this task because noninvasive retinal imaging technology is rapidly advancing and can provide a detailed assessment of the retinal vasculature. Structural and functional retinal vascular changes have been associated with cardiovascular disease as well as other. systemic diseases.
The workshop identified several roadblocks that must be addressed in order to facilitate the development of retinal imaging biomarkers of cardiovascular disease. One key challenge is the need to simplify and standardize the process for obtaining high quality images, especially by technicians and clinicians in non-ophthalmic settings. Another challenge is the lack of longitudinal studies in diverse participant groups - the large datasets needed for this sort of analysis can be complicated by ethical and patient privacy concerns. Many other research gaps were identified, including improving the measurement of structural and functional biomarkers and improved standardization of the imaging devices and protocols.
In addition, more research into the relationship between microvascular and macrovascular risk factors is critical, and there is increasing interest in combining retinal biomarker data with other data such as genetics and lifestyle-related risk factors using artificial intelligence approaches (see Figure).
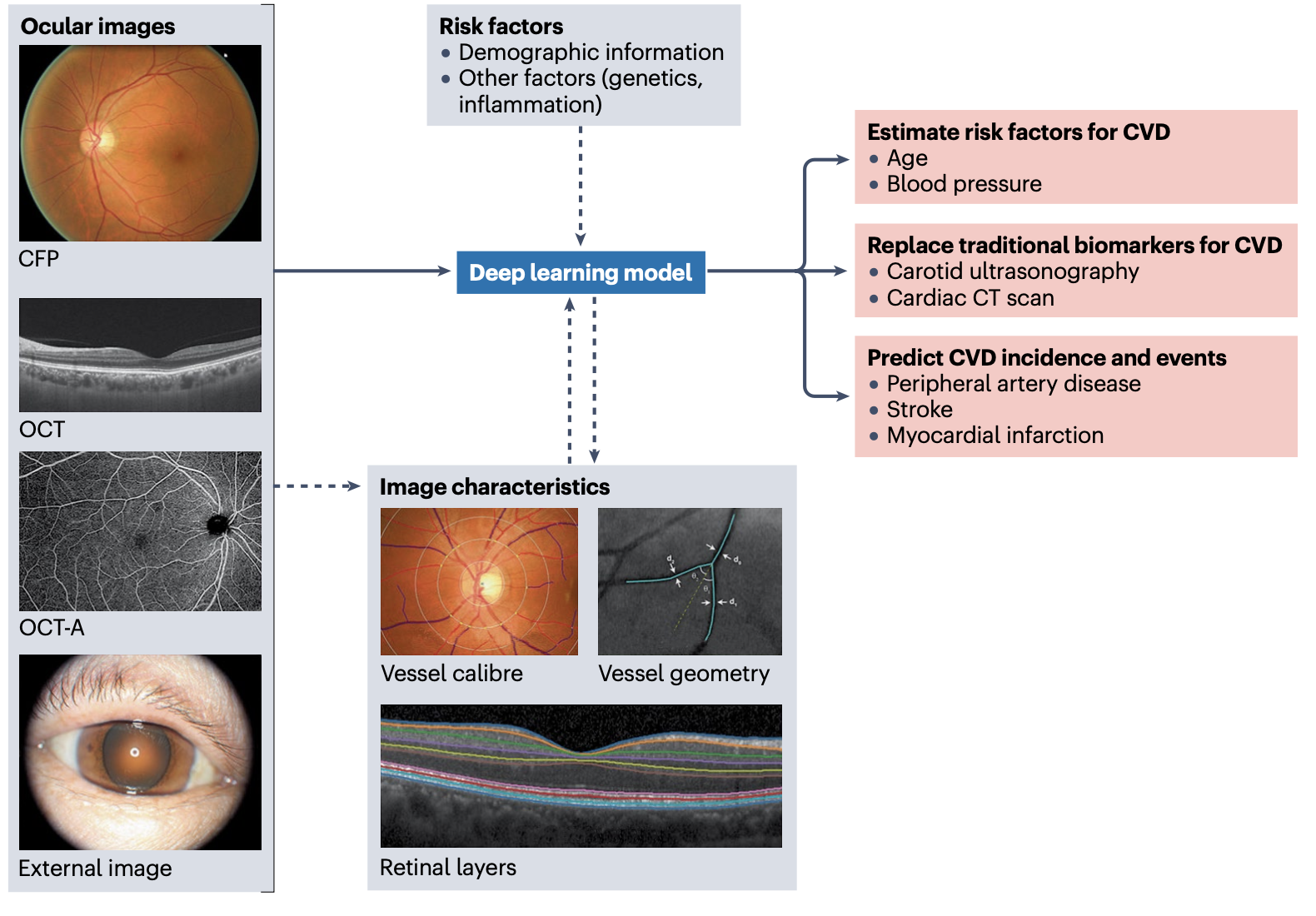
Application of AI to retinal images.
Ocular images, such as colour fundus photographs (CFPs), optical coherence tomography (OCT) and OCT angiography (OCT-A) scans, and external photographs of the eye are fed into deep learning models, either independently or together with information on other relevant risk factors to increase the predictive accuracy. Advanced image analysis techniques and deep learning models can extract meaningful features from these ocular images (such as vessel calibre, vessel geometry and retinal layers), which can also be fed into the prediction models. The deep learning models can then be used to estimate risk factors associated with cardiovascular disease (CVD), potentially replacing the use of traditional biomarkers of CVD derived from more expensive imaging of the cardiovascular system. Moreover, deep learning models can be used to predict the risk of specific CVD events. Adapted from ref. 132, Springer Nature Limited.
Future directions identified in the workshop include developing the strategy for clinical implementation of retinal vessel analysis in clinical practice, encouraging interdisciplinary collaboration, the development of networks and consortia to help facilitate such collaboration, and the development of a centralized data repository to enable the sharing of resources while building a strong evidence base. Going forward, the hope is that simple noninvasive eye imaging will be widely accepted as a key window into systemic health, providing clinicians with another tool to monitor their patients overall health.