In this paper, published in JCI Insight, the authors describe their deep learning model for quantifying vaso-obliteration and neovascularization on retinal images, which are key measurements in oxygen-induced retinopathy mouse model studies of ischemia-driven neovascularization. The mouse model of oxygen-induced retinopathy is one of the most commonly used in vivo models to study basic mechanisms in ocular angiogenesis and to test potential therapeutics. Measurements of vaso-obliteration and neovascularization are often used in proof-of-concept studies evaluating antiangiogenic drugs for diseases such as age-related macular degeneration and diabetic retinopathy.
Deep learning is a subfield of machine learning in which feature construction (building intermediate "features" from the descriptors in the data) is automated and learned implicitly through a purely data-driven approach using many layers of neural networks. By using a deep learning approach, the authors were able to address some of the key problems with evaluating images from the oxygen-induced retinopathy mouse model. Reproducibility of experimental results can be a major challenge due to high variability in the vascular phenotype, and manual analysis is time-consuming and requires expertise.
The model identified and segmented the areas of vaso-obliteration and neovascularization in the oxygen-induced retinopathy images with high correlation to 4 separate expert human graders. In addition, the model identified the area of total retina in a fully automated fashion, without the need for subjective manual input. The algorithm processed the images quickly, making it scalable to many hundreds or thousands of OIR images, and can run on a standard desktop computer without the need for specialized hardware.
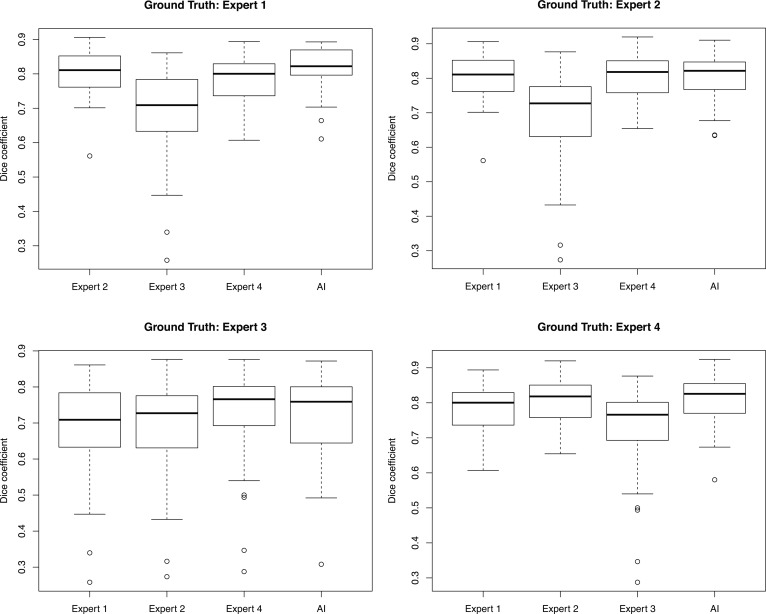
This model should prove to be very helpful for researchers who use the OIR mouse model to evaluated candidate drug therapies. Automating the analysis of the OIR images should improve efficiency and the overall quality the results, and may even allow for detection of more subtle changes in the images in the future. When labs use this tool, they will automatically apply the same standards for the identification of vaso-obliteration and neovascularization as other labs that use it, thereby increasing the generalizability and reproducibility of their study results.
To summarize, this software tool enables researchers to avoid a repetitive and time-consuming manual quantification process, and allows for better comparisons between studies. The authors note that future extensions of this tool could include a web-based segmentation system in which experts could provide corrected feedback for online iterative training of the deep learning models.
Xiao S, Bucher F, Wu Y, Rokem A, Lee CS, Marra KV, Fallon R, Diaz-Aguilar S, Aguilar E, Friedlander M, Lee AY. Fully automated, deep learning segmentation of oxygen-induced retinopathy images. JCI Insight. 2017 Dec 21;2(24). doi: 10.1172/jci.insight.97585. PubMed PMID: 29263301; PubMed Central PMCID: PMC5752269.