In this commentary for the journal Translation Vision Science and Technology, Dr. Aaron Lee and Dr. Cecilia Lee discuss how the application of artificial intelligence and big data in healthcare has the potential to transform clinical trial research.
They explain some of the common pitfalls of randomized controlled trials and how deep learning is particularly well-suited to overcome some of these problems, leading to more efficient execution and greater statistical power than what would be expected from traditional trials.
For example, machine learning can be used to combine different types of data, such as imaging, laboratory, and other complex -omics data, to screen and select patients who match complex inclusion criteria, which can help to make patient recruitment more efficient. Machine learning can also be used to optimize the effect size of a treatment or intervention, which could be especially useful for the development of novel therapies, in particular for rare diseases.
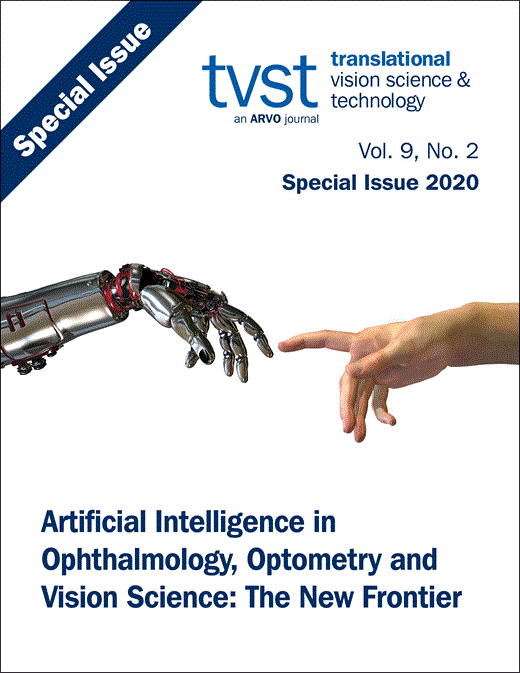
Other potential benefits of machine learning in clinical trials include minimizing measurement errors, reducing bias, creating more sensitive quantification of key study endpoints, generating new endpoints, and measuring treatment effects. With sufficient data, artificial intelligence models may even allow the use of a synthetic control arm in the future. By training a model to predict the natural history of each participant, substitution of the control arm by virtual controls may be possible, which could reduce the number of participants needed by a significant amount.
The authors note that large scale collaboration is needed to enable the storage of well-curated datasets, and that measurement error and the "black box" issue are potential problems. They suggest that small steps such as creating an "AI arm" alongside the usual study and control arms in future randomized controlled trials would be useful for exploring the potential of machine learning approaches. Randomized controlled trials are the gold standard for measuring the effectiveness of new treatments. Artificial intelligence approaches will not replace these trials but they have the potential to significantly improve and complement them in the future.
Lee CS, Lee AY. Clinical applications of continual learning machine learning. Lancet Digital Health 2 (6), e279-e281, 2020.