In this article published in the journal Diabetes Care, Dr. Aaron Lee and his co-authors put diabetic retinopathy screening algorithms to the test in the real world, evaluating them on retinal images from nearly 24,000 veterans who sought diabetic retinopathy screening at two Veterans Affairs health care systems (Seattle and Atlanta). These screening algorithms are designed to check patients who might be at risk for retinopathy, a potential complication of diabetes that can lead to blindness if left untreated. Based on performance in clinical trials, one of these algorithms is approved for use in the US, and several others are in clinical use in other countries. Dr. Lee wanted to know how well they worked outside of the clinical trial setting, however, when faced with real world data from a diverse group of patients in a variety of clinical settings.
Five companies submitted a total of seven algorithms for this study. First the algorithms were evaluated on how accurately they picked up signs of diabetic retinopathy by comparing their results to those of human teleretinal screeners, who normally perform this screening task at VA hospitals. Next the researchers gave a subset of the images to expert ophthalmologists for evaluation, and the decisions from both the algorithms and the human graders were then compared to these expert opinions.
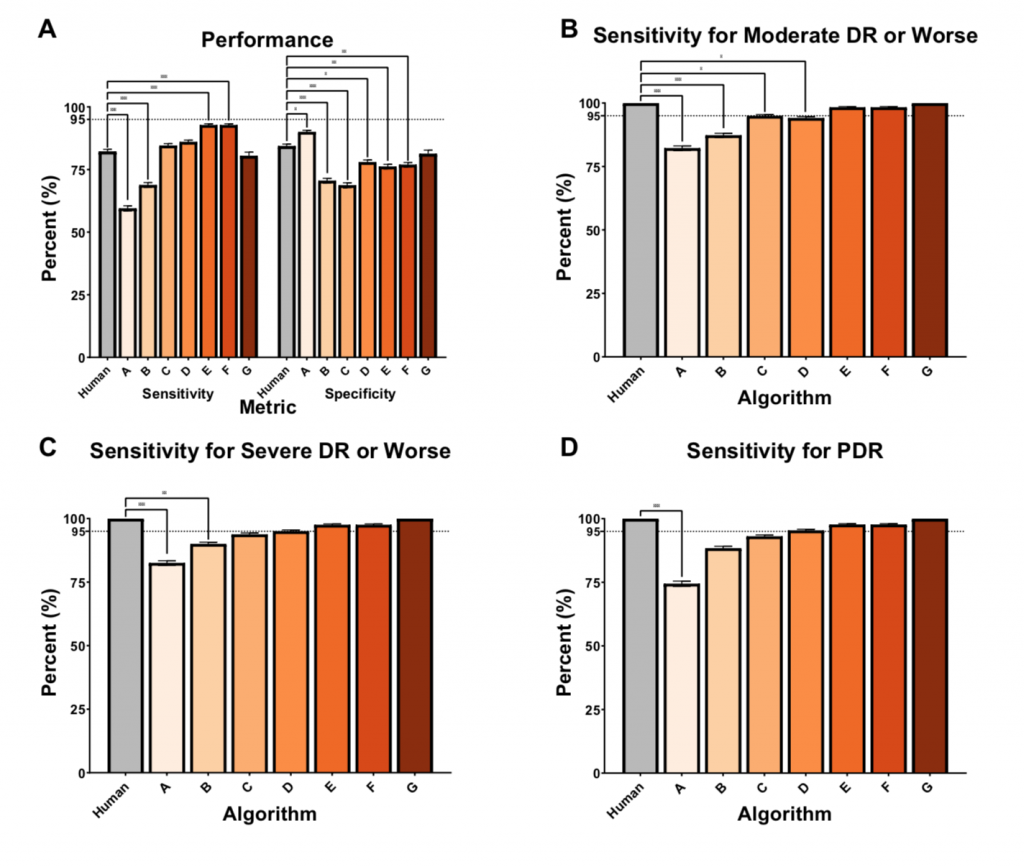
Relative performance of human grader compared to AI algorithms. The relative performance of the Veterans Affairs (VA) teleretinal grader (Human) and Algorithms A-G in screening for referable diabetic retinopathy (DR) using the arbitrated dataset at different thresholds of diabetic retinopathy (DR). (A) shows sensitivity and specificity of each algorithm compared to a human grader with 95% confidence interval bars against a subset of double, masked arbitrated grades, in screening for referable DR in images with mild nonproliferative DR (NPDR) or worse and ungradable image quality. In (B-D), only gradable images were used. The VA teleretinal grader is compared to the AI sensitivities, with 95% confidence intervals, at different thresholds of disease, including moderate NPDR or worse in (B), severe NDPR or worse in (C), and proliferative DR (PDR) in (D). *, p ≤ 0.05, ** p ≤ 0.001; *** p ≤ 0.0001.
Surprisingly, the algorithms did not perform as well as might have been expected. Three of the algorithms performed reasonably well when compared to the expert diagnoses and one did worse - worrisomely, it was less sensitive for the more severe case of proliferative diabetic retinopathy than the human screeners. And only one algorithm performed as well as the human screeners in the test.
Interestingly, the algorithms performed differently in the two patient populations (Seattle and Atlanta), which could be related to variationss in disease prevalence and demographics between the two patient populations. This suggests that it may be important to test these algorithms in the settings in which they will be used, to ensure that they perform as intended.
A strength of this study was that the researches were able to report the negative predictive value for each algorithm, or how often the algorithm states that there is no disease when patients actually do not have disease. This can be difficult to measure but is an essential assessment of their performance, since the main goal for these algorithms is that they do not let a single patient with diabetic retinopathy slip through the screening system.
This study highlights some of the significant real world considerations that may impact the use of artificial intelligence devices as they are increasingly incorporated into the clinical workflow. These devices show great promise to assist physicians with their clinical decision-making, but it is essential that clinicians, regulators, and patients understand how the algorithms work in a particular setting and what factors may affect their performance.
Lee AY, Yanagihara RT, Lee CS, Blazes M, Jung HC, Chee YE, Gencarella MD, Gee H, Maa AY, Cockerham GC, Lynch M, Boyko EJ. Multicenter, Head-to-Head, Real-World Validation Study of Seven Automated Artificial Intelligence Diabetic Retinopathy Screening Systems. Diabetes Care. 2021 Jan 5;. doi: 10.2337/dc20-1877. [Epub ahead of print] PubMed PMID: 33402366