In this study, Dr. Aaron Lee, Dr. Cecilia Lee and their co-authors used a unique artificial intelligence approach for identifying novel anatomic biomarkers in the retinas of patients with age-related macular degeneration. Patients with this condition can progressively lose their central vision, and there are still no effective treatments for the more common dry (non exudative) form of the disease. Delayed rod-mediated dark adaptation is the method through which the retina recovers after a stimulus of bright light, and this process can be measured as a function of time to help predict which patients are at risk for age-related macular degeneration and vision loss. The authors wanted to take advantage of the increasingly advanced retinal imaging technology available to search for structural changes in the retina that might be associated with dark adaptation dysfunction, to better understand the cellular and structural pathology that occurs early the in age-related macular degeneration disease process.
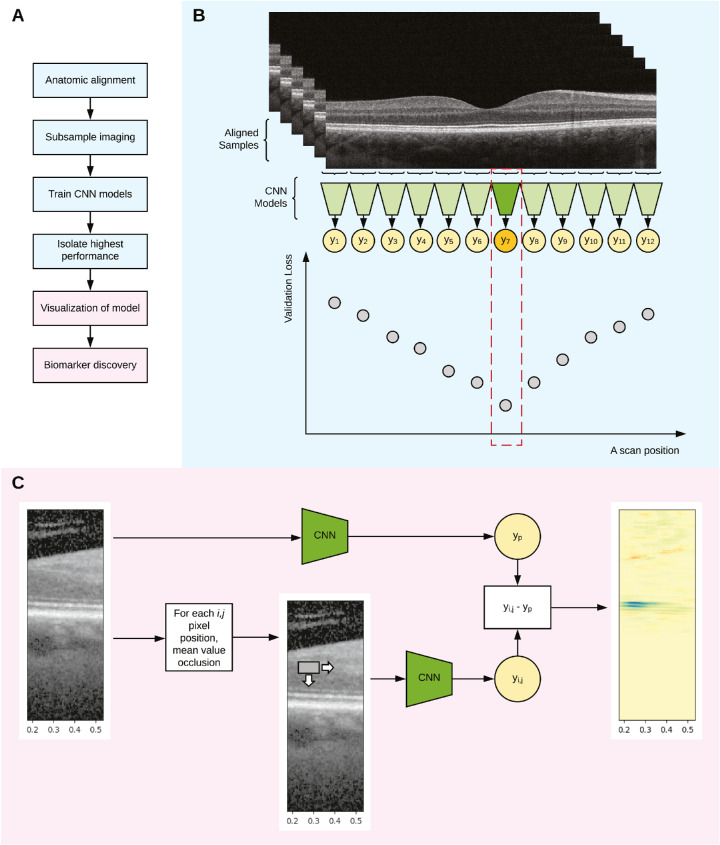
The authors created a deep learning model that analyzed optical coherence tomography images from patients with different stages of disease. They trained the model to predict rod-mediated dark adaptation status from the images. The model learned to accurately predict a patient's "rod intercept time", or the time it took for their retina to recover from a bright light stimulus, by analyzing retinal structural changes in the images. To determine which anatomic regions were associated with dark adaptation dysfunction, the authors first restricted the model to various discrete anatomical regions, and then determined which models performed best. Then they used a technique called occlusion testing to narrow down the region of interest even further. Using this approach, the authors were able to identify two areas of hyporeflective bands that were associated with decreased dark adaption function.
These two hyporeflective bands in the optical coherence tomography images of the retina represent the myoid zone of the photoreceptors and a region between the ellipsoid zone and the interdigitation zone. The myoid zone is known to shorten in age-related macular degeneration, and the other hyporeflective region is also of interest because it contains structures that help further explain some of the complicated mechanisms associated with dark adaptation specifically and age-related macular degeneration more broadly.
This work is novel in that the deep learning model was able to agnostically identify anatomic regions associated a known functional measure of early age-related macular degeneration. This technique could be used to identify biomarkers in other retinal disorders, and have to the potential to help further our understanding of the pathophysiological mechanisms involved at a cellular level.
Lee AY, Lee CS, Blazes MS, Owen JP, Bagdasarova Y, Wu Y, Spaide T, Yanagihara RT, Kihara Y, Clark ME, Kwon M, Owsley C, Curcio CA. Exploring a Structural Basis for Delayed Rod-Mediated Dark Adaptation in Age-Related Macular Degeneration Via Deep Learning. Transl Vis Sci Technol. 2020 Dec;9(2):62. doi: 10.1167/tvst.9.2.62. eCollection 2020 Dec. PubMed PMID: 33344065; PubMed Central PMCID: PMC7745629.